|
|
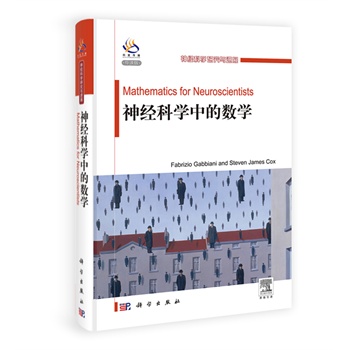 |
《神经科学中的数学(导读版)》 |
作者:(美)伽比阿尼 等编著 |
出版社:科学出版社 |
出版日期:2012/1/1 |
ISBN:9787030331144 |
定价: 135.00元 |
|
编辑推荐
在神经科学的研究中,大量数学方法被用于表述已知原理和分析实验数据。《神经科学研究与进展:神经科学中的数学(英文)(导读版)》广泛阐述了数学、神经科学及二者交叉领域的基础原理,引导初学者逐步理解和掌握数学方法在神经科学中的应用。各章节交替介绍数学概念和数值计算方法,以及这些概念和方法在神经科学研究中的应用实例,涉及领域涵盖传统的细胞生物物理学研究和系统水平的神经生物学研究。数学知识循序渐进,深度递增,便于非数学专业的读者理解掌握。每章附有大量练习题及解答,内容均衍生于撰稿人的课程教材。每种计算模型包括MATLAB编码,方便读者使用。《神经科学研究与进展:神经科学中的数学(英文)(导读版)》属于通用工具,可以帮助神经生物学研究者采用合理的数据处理和统计方法得出结论。简明易懂,适合初学者和相关领域的研究人员作为参考书。
《神经科学研究与进展:神经科学中的数学(英文)(导读版)》特点:对线性代数、傅立叶变換、常微分和偏微分方程、概率论、随机过程和信号处理理论进行数学和计算方面的系统介绍,阐述亚细胞、细胞和系统神经科学水平等多课题的基础,大暈的练习题和解决方案辅助网站提供232个模拟结果的Matlab源代码。
内容推荐
《神经科学研究与进展:神经科学中的数学(英文)(导读版)》通过Matlab编程语言在众多模拟中的应用来介绍计算方法。这些程序为新的课程和研究提供有益的跳板。作者从介绍微分方程和线性代数在细胞、亚细胞和突起模型的应用开始,然后介绍概率论在突触传递和单细胞噪声中的应用,最后将信号处理理论应用于系统神经科学中。神经科学依赖众多数学工具表达已有的理论、分析数据并提出新的实验。《神经科学研究与进展:神经科学中的数学(英文)(导读版)》采用一系列扎实的计算模型将该领域最令人瞩目的工具由浅入深地介绍给读者。旨在为神经科学专业的本科生和研究生,以及对神经科学感兴趣的数学、物理和工程背景的学生提供一本教科书,亦可为进行神经科学相关研究的工作者提供有用的参考。
目录
前言
1.简介
1.1如何使用本书
1.2大脑简介
1.3基础数学知识
1.4计量单位
1.5参考资料来源
2.被动等电位细胞
2.1简介
2.2能斯特电位
2.3膜电导
2.4膜电容与电流平衡
2.5突触电导
2.6总结与参考资料来源
2.7练习题
3.微分方程
3.1精确求解
3.2矩量法
3.3拉普拉斯变换
3.4数值分析法
3.5突触输入
3.6总结与参考资料来源
3.7练习题
4.主动等电位细胞
4.1延迟整流钾离子通道
4.2钠离子通道
4.3Hodgkin-Huxley公式
4.4瞬时钾离子通道
4.5总结与参考资料来源
4.6练习题
5.半主动等电位细胞
5.1半主动模型
5.2数值分析法
5.3特征向量扩展的精确求解
5.4持续钠电流
5.5超极化激活的非特异性阳离子电流
5.6总结与参考资料来源
5.7练习题
6.被动电缆理论
6.1离散的被动电缆公式
6.2特征向量扩展的精确求解
6.3数值分析法
6.4被动电缆公式
6.5突触输入
6.6总结与参考资料来源
6.7练习题
7.傅立叶级数与变换
7.1傅立叶级数
7.2离散的傅立叶变换
7.3连续傅立叶变换
7.4协调离散与连续傅立叶变换
7.5总结与参考资料来源
7.6练习题
8.被动树突野
8.1离散的被动树突野
8.2特征向量扩展
8.3数值分析法
8.4被动树突公式
8.5(树突电缆的)等效圆柱体
8.6(树突)分支的特征函数
8.7总结与参考资料来源
8.8练习题
9.主动树突野
9.1主动的均一电缆
9.2主动均一电缆的相互作用
9.3主动非均一电缆
9.4半主动电缆
9.5主动树突野
9.6总结与参考资料来源
9.7练习题
10.简化的单神经元模型
10.1漏电的整合一发放神经元
10.2簇放电神经元
10.3簇放电神经元的简化模型
10.4总结与参考资料来源
10.5练习题
11.概率与随机变量
11.1事件与随机变量
11.2符合二项式分布的随机变量
11.3符合泊松分布的随机变量
11.4符合高斯分布的随机变量
11.5累计分布函数
11.6条件概率
11.7独立随机变量的加和
11.8随机变量的变换
11.9随机向量
11.10指数与伽玛分布的随机变量
11.11齐次泊松过程
11.12总结与参考资料来源
11.13练习题
12.突触传递与量子释放理论
12.1突触的基本结构与生理
12.2量子释放的发现
12.3突触释放的复合泊松模型
12.4与实验数据的对比
12.5中枢系统突触的量子分析
12.6突触传递的易化、增强与抑制
12.7短时程突触可塑性的模型
12.8总结与参考资料来源
12.9练习题
13.神经元钙流信号
13.1电压门控型钙离子通道
13.2胞内钙离子的扩散、缓释与再摄取
13.3电镜所揭示的钙离子释放
13.4树突小棘的钙离子
13.5突触前钙离子和神经递质释放
13.6总结与参考资料来源
13.7练习题
14.奇异值分解算法及其应用
14.1奇异值分解算法
14.2主成分分析与动作电位发放的归类
14.3突触可塑性与主成分
14.4通过均衡截断方法精简神经元模型
14.5总结与参考资料来源
14.6练习题
15.动作电位发放波动的定量分析
15.1动作电位发放的时间间距柱形统计图与变异系数
15.2动作电位不应期
15.3动作电位发放数分布与法诺因子
15.4更新过程
15.5返回图与经验相关因子
15.6总结与参考资料来源
15.7练习题
16.随机过程
16.1定义与一般特性
16.2高斯过程
16.3点过程
16.4非均一泊松过程
16.5频谱分析
16.6总结与参考资料来源
16.7练习题
17.膜噪声
17.1两状态通道模型
17.2多状态通道模型
17.3Ornstein-Uhlenbeck过程
17.4突触噪声
17.5总结与参考资料来源
17.6练习题
18.能量谱与互相关分析
18.1互相关与互相干
18.2估计量偏倚与方差
18.3能量谱的数值估计
18.4总结与参考资料来源
18.5练习题
19.自然光信号与光(电)转导
19.1波长与光强
19.2自然光信号的空间特性
……
20.(动作电位)发放率编码与早期视觉
21.简单细胞与复杂细胞模型
22.随机估计理论
23.反相关分析与动作电位发放解码
24.信号检测理论
25.神经元反应与心理物理学的关联研究
26.群体编码
27.神经元网络
28.练习题解答
参考文献
索引
在线试读部分章节
1
Introduction
O U T L I N E
1.1 How to Use this Book 2
1.2 Brain Facts Brief 2
1.3 Mathematical Preliminaries 4
1.4 Units 7
1.5 Sources 8
Faced with the seemingly limitless qualities of thebrain,Neuroscience has eschewed provincialism and instead
pursued a broad tack that openly draws on insightsfrombiology,physics,chemistry,psychology,and mathematics in
its construction of technologies and theories with which toprobe and understand the brain.These technologies and
theories,in turn,continue to attract scientists andmathematicians to questions of Neuroscience.As a result,we may
trace over one hundred years of fruitful interplay betweenNeuroscience and mathematics.This text aims to prepare
the advanced undergraduate or beginning graduate student to takean active part in this dialogue via the application
of existing,or the creation of new,mathematics in the interestof a deeper understanding of the brain.Requiring no
more than one year of Calculus,and no prior exposure toNeuroscience,we prepare the student by
1.introducing mathematical and computational tools in preciselythe contexts that first established their importance
for Neuroscience and
2.developing these tools in concrete incremental steps within acommon computational environment.
As such,the text may also serve to introduce Neuroscience toreaders with a mathematical and/or computational
background.
Regarding (1),we introduce ordinary differential equations viathe work of Hodgkin and Huxley (1952) on action
potentials in the squid giant axon,partialdifferential equationsthroughthework ofRall oncable theory (see Segev et al.
(1994)),probability theory following the analysis of Fatt andKatz (1952) on synaptic transmission,dynamical systems
theory in the context of Fitzhugh's (1955) investigation ofaction potential threshold,and linear algebra in the context
of the work of Hodgkin and Huxley (1952) on subthresholdoscillations and the compartmental modeling of Hines
(1984) on dendritic trees.In addition,we apply Fouriertransforms to describe neuronal receptive fields following
Enroth-Cugell and Robson's (1966) work on retinal ganglion cellsand its subsequent extension toHubel andWiesel's
(1962) characterization of cat cortical neurons.We alsointroduce and motivate statistical decision methods starting
with the historical photon detection experiments of Hecht etal.(1942).
Regarding (2),we develop,test,and integratemodels ofchannels,receptors,membranes,cells,circuits and sensory
stimuli by working from the simple to the complex within theMATLAB computing environment.Assuming no prior
exposure toMATLAB,we develop and implement numerical methods forsolving algebraic and differential equations,for computing Fourier transforms,and for generating andanalyzing random signals.Through an associatedweb site
we provide the studentwithMATLAB code for 144computationalfigures in the text andwe provide the instructorwith
MATLAB code for 98 computational exercises.The exercises rangefrom routine reinforcement of concepts developed
in the text to significant extensions that guide the reader tothe research literature.Our reference to exercises both in
the text and across the exercises serve to establish them as anintegral component of this book.
Concerning themathematical models considered in the text,we citethe realization of Schr?dinger (1961) that "we
cannot ask for more than just adequate pictures capable ofsynthesizing in a comprehensible way all observed facts
and giving a reasonable expectation on new oneswe are outfor."Furthermore,lest "adequate"serve as an invitation
to loose or vague modeling,Schr?dinger warns that "without anabsolutely precise model,thinking itself becomes
imprecise,and the consequences derived from the model becomeambiguous.”
As we enter the 21st century,one of the biggest challengesfacing Neuroscience is to integrate knowledge and to
craft theories that span multiple scales,both in space from thenanometer neighborhood of an ion channel to the
meter that action potentialsmust travel down the sciaticnerve,and in time from the fraction of a millisecond it takes
to release neurotransmitter to the hours it takes to prune orgrow synaptic contacts between cells.We hope that this
text,by providing an integrated treatment of experimental andmathematical tools within a single computational
framework,will prepare our readers to meet this challenge.
1.1 HOW TO USE THIS BOOK
The book is largely self-contained and as such is suited forboth self-study and reference use.The chapters need not
be read in numerical order.To facilitate a selection forreading,we have sketched in Figure 1.1 themain dependencies
between the chapters.The four core chapters that underlie muchof the book are Chapters 2-4 and 11.For the reader
with limited prior training in mathematics it is in thesechapters that we develop,by hand calculation,MATLAB
simulation and a thorough suite of exercises,the mathematicalmaturity required to appreciate the chapters to come.
Many of the basic chapters also contain more advancedsubsections,indicated by an asterisk,?,which can be skipped
on a first reading.Detailed solutions are provided for mostexercises,either at the end of the book or through the
associatedweb site.We mark with a dagger,?,each exercise whosesolution is not included in this text.
Over the past eight years,we have used a subset of the book'smaterial for a one semester introductory course
on Mathematical Neuroscience to an audience comprised of Scienceand Engineering undergraduate and graduate
students from Rice University and Neuroscience graduate studentsfrom Baylor College of Medicine.We first cover
Chapters 2-5,which set and solve the Hodgkin-Huxley equationsfor isopotential cells and,via the eigenvector
expansion of the cell's subthreshold response,introduce the keyconcepts of linear algebra needed to tackle the
multicompartment cell in Chapters 6 and 8-9.We then open Chapter11,introduce probabilistic methods and apply
them to synaptic transmission,in Chapter 12,and spike trainvariability,in Chapter 15.We conclude this overview
of single neuron properties by covering Chapter 10 on reducedsingle neuron models.We transition to Systems
Neuroscience via the Fourier transform of Chapter 7 and itsapplication to visual neurons in Chapters 20 and 21.
Finally,we connect neural response to behavior via thematerialof Chapters 24 and 25.An alternative possibility is to
conclude withChapters 22 and 23,after an informal introductionto stochastic processes,and power and cross spectra
in Chapters 16 and 18.
We have also used the following chapters for advanced courses:13,14,16-19,and 26.Chapter 13 provides a
comprehensive coverage of calcium dynamics within single neuronsat an advanced level.Similarly,Chapter 14
introduces the singular value decomposition,a mathematical toolthat has important applications both in spike
sorting and in model reduction.Chapters 16 and 18 introducestochastic processes and methods of spectral analysis.
These results can be applied at the microscopic level todescribe single channel gating properties,Chapter 17,and at
the macroscopic level to describe the statistical properties ofnatural scenes and their impact on visual processing,Chapter 19.Finally the chapters on population codes andnetworks,Chapters 26 and 27,address the coding and
dynamical properties of neuronal ensembles.
To ease the reading of the text,we have relegated all referencesto the Summary and Sources section located at the
end of each chapter.These reference lists are offered aspointers to the literature and are not intended to beexhaustive.
1.2 BRAIN FACTS BRIEF
The brain is the central component of the nervous system and isincredibly varied across animals.In vertebrates,it
is composed of threemain subdivisions: theforebrain,themidbrain,and the hindbrain.Inmammals andparticularly
in humans,the cerebral cortex of the forebrain is highlyexpanded.The human brain is thought to contain on the
order of 100 billion (1011) nerve cells,or neurons.Each neuron"typically"receives 10,000 inputs (synapses,§2.1)
from other neurons,but this number varies widely across neurontypes.For example: granule cells of the cerebellum,the most abundant neurons in the brain,receive on average fourinputs while Purkinje cells,the output neurons of
the cerebellar cortex,receive on the order of 100,000.In themouse cerebral cortex,the number of neurons per cubic
millimeter has been estimated at 105,while there areapproximately 7×108 synapses and 4 km of cable (axons,§2.1)
in the same volume.Brain size (weight) typically scales withbody size,thus the human brain is far from the largest.
At another extreme,the brain of the honey bee is estimated tocontain less than a million (106) neurons within a
single cubic millimeter.Yet the honey bee can learn a variety ofcomplex tasks,not unlike those learned by a macaque
monkey for instance.Although it is often difficult to drawcomparisons across widely different species,the basic
principles underlying information processing as they arediscussed in this book appear to be universal,in spite of
obvious differences in implementation.The electrical propertiesof cells (Chapter 2),the generation and propagation
of signals along axons (Chapters 4 and 9),and the detection ofvisual motion (Chapters 21 and 25) or population codes
(Chapter 26),for instance,are observed to follow very similarprinciples across very distantly related species.
Information about the environment reaches the brain through fivecommon senses: vision,touch,hearing,smell,and taste.In addition,some animals are able to sense electricfields through specialized electroreceptors.These include
many species of fish andmonotremes (egg-layingmammals) like theplatypus.Most sensory information is gathered
from the environment passively,but some species are able to emitsignals and register their perturbation by the
environment and thus possess active sensory systems.Thisincludes bats that emit sounds at specific frequencies and
hear the echoes bouncing off objects in the environment,aphenomenon called echolocation.In addition some species
of fish,termedweakly electric,possess an electric organ allowingthem to generate an electric field around their body
and sense its distortion by the environment,a phenomenon calledelectrolocation.
Ultimately,the brain controls the locomotor output of theorganism.This is typically a complex process,involving
both commands issued to the muscles to executemovements,feedback from sensors reporting the actual state ofthe
musculature and skeletal elements,and inputs from the senses tomonitor progress towards a goal.So efficient is this
process that even the tiny brain of a fly is,for instance,ableto process information sufficiently fast to allow for highly
acrobatic flight behaviors,executed in less than 100 ms fromsensory transduction to motor output.
To study the brain,different biological systems have provenuseful for different purposes.For example,slices of the
rat hippocampus,a structure involved in learning and memory aswell as navigation,are particularly adequate for
electrophysiological recordings of pyramidal neurons and adetailed characterization of their subcellular properties,because their cell bodies are tightly packed in a layer that iseasy to visualize.The fruit fly Drosophila melanogaster and
thewormCaenorhabditis elegans (whosenervous systemcomprisesexactly 302 neurons) are goodmodels to investigate
the relation between simple behaviors and genetics,as theirgenomes are sequenced and many tools are available to
selectively switch on and off genes in specific brain structuresor neurons.One approach that has been particularly
successful to study informationprocessing in the brain is"neuro-ethological,"based on the study of natural behaviors
(ethology) in relation to the brain structures involved in theirexecution.Besides the alreadymentionedweakly electric
fish and bats,classical examples,among many others,include songlearning in zebrafinches,the neural control of
flight in flies,sound localization in barn owls,and escapebehaviors in a variety of species,such as locust,goldfish,or flies.
1.3 MATHEMATICAL PRELIMINARIES
MATLAB.Native MATLAB functions are in typewriterfont,e.g.,svd.Our contributed code,available on the book's
web site,has a trailing .m,e.g.,bepswI.m.
Numbers.The counting numbers,{0,1,2,...},are denoted by N,whilethe reals are denoted by R and the complex
numbers by C.Each complex number,z∈C,may be decomposed into itsreal and imaginary components.Wewillwrite
z=x+iy,where x=(z),y=(z),and i ≡ √?1.
Here x and y are each real and ≡ signifies that one side isdefined by the other.We denote the complex conjugate and
magnitude of z by
z? ≡x?iy and |z|≡x2+y2,respectively.
Sets.Sets are delimited by curly brackets,{}.For example the setof odd numbers between 4 and 10 is {5,7,9}.
Intervals.For a,b∈R with a
[a,b] is the set of numbers x such that a≤x≤b.The semiclosed (orsemiopen) intervals [a,b) and (a,b] are the set of
numbers x such that a≤x
Vectors and matrices.Given n real or complexnumbers,x1,x2,...,xn,we denote their arrangement into avector,or
column,via bold lower case letters,x=x1x2...xn
.(1.1)
The collections of all real and complex vectors with ncomponents are denoted Rn and Cn,respectively.The transpose
of a vector,x,is the row,xT =(x1 x2…xn),and the conjugate transpose of a vector,z∈Cn,is the row
zH =(z?1 z?2…z?n).
We next define the inner,or scalar,or "dot,"product for x and yin Cn,xHy≡nj=1
x?j yj,and note that as
zHz=i=1
|zi|2 ≥0
MATHEMATICS FOR NEUROSCIENTISTS
it makes sense to define the norm
z
≡
zHz.
To gain practice with these definitions you may wish to confirmthat
(yHy)x?(yHx)y
2=
y
2(
y
2
x
2?|xHy|2).
As the left hand side is nonnegative the right hand side revealsthe important Schwarz inequality
|xHy|≤
x
y
.(1.2)
We will transform vectors in Cn to vectors in Cm viamultiplication by m×n matrices,A∈Cm×n,of the form
A=?????
A11 A12…A1n
A21 A22…A2n
Am1 Am2…Amn
?????
where each Aij ∈C.Thus,y=Ax means that yi =nj
=1Aijxj for i=1,...m.Wewill consistently denotematrices bybold
upper case letters.Given A∈Cm×n and B∈Cn×p we define theirproduct C∈Cm×p via
C=AB where Cjk =
n
l=1
AjlBlk .
If we reflect A about its diagonal we arrive at its transpose,AT∈Cn×m,where (AT)ij =Aji.The associated conjugate
transpose is denoted AH,where (AH)ij =A?ji.We will often requirethe conjugate transpose of the product AB,and so
record
(AB)H
jk =nl=1
A?klB?lj =(BHAH)jk,i.e.,(AB)H =BHAH.(1.3)
Similarly,(AB)T =BTAT.
The identity matrix,denoted I,is the square matrix of zeros offthe diagonal,and ones on the diagonal,We often use the Kronecker delta
δjk ≡1,if j=k
0,otherwise
(1.4)
to denote the elements of I.Amatrix B∈Cn×n is said to beinvertible if there exists a matrix B?1 ∈Cn×n such that
BB?1=B?1B=I.(1.5)
In this case B?1 is called the inverse of B.
MATHEMATICS FOR NEUROSCIENTISTS
Functions.We will make frequent use of the characteristicfunction
1(a,b)(x)≡1,if a
0,otherwise
(1.6)
of the interval,(a,b).In the common case that (a,b) is the setof nonnegative reals we will simply write 1(x) and refer
to it as the Heaviside function.
We will often need to differentiate the running integral,F(x)=
x
0f (y)dy.
To see that
F(x)=f (x) (1.7)
when f is continuous at x,note that the mean value theoremestablishes the second equality in
F(x+h)?F(x)
h =1hx+h
x
f (y)dy=f (xh) (1.8)
for some xh ∈(x,x+h).As h→0 the left hand side approaches F(x)while on the right xh→x and so,by continuity,f (xh)→f (x).
We will often need to sample,or discretize,scalar valuedfunctions,f :R→R,of time and/or space.For example,if time is divided into increments of size dt then we willdenote the samples of f (t) by superscripted letters in the
"typewriter"font
fj ≡f (( j?1)dt),j=1,2,3,....
Similarly,we will denote the samples of a vector valuedfunction,f :R→Rn,by superscripted letters in the bold
typewriter font
fj ≡f(( j?1)dt),j=1,2,3,....
The elements of fj are samples of the elements of f.We expressthis in symbols as fj
m=fm(( j?1)dt).Where the
superscript,j,may interfere with exponents we will be careful tomake the distinction.
Random variables.In chapters dealing with random variables,wewill try whenever possible to use upper case
letters for a random variable and lower case letters for aspecific value of the same random variable.We denote the
expectation or mean of a random variable X by E[X].The varianceis the expectation of the squared deviation of
X from the mean: E[(X?E[X])2].A Gaussian or normal randomvariable of mean μ and variance σ2 is denoted by
N(μ,σ2 ).An estimator of,e.g.,the mean mX of a random variable Xis denoted by m? X.
Fourier transforms.The Fourier transform of a function f (t) oftime,t,is denoted by ?f (ω):
?f (ω)≡
∞
?∞
f (t)e?2πiωtdt.
The variable ω is the ordinary frequency.If t has units ofseconds (s) then ω has units of 1/s=Hz (Hertz).The
convolution of two functions f and g is denoted by fg:
( f g)(t)≡
∞?∞
f (t1)g (t?t1)dt1.(1.9)
|
|
|